AI revolutionizes drug discovery market, projected to reach $7.94 billion by 2030
[Artificial Intelligence. Photo Credit to Pixabay]
The AI-driven drug discovery market is projected to grow from $3 billion in 2022 to $3.54 billion in 2023, eventually reaching $7.94 billion by 2030, according to a February 2025 report from Fortune Business Insights.
This AI-driven technology is driving innovation by reducing costs, shortening research timelines, and improving success rates in drug development.
The use of AI in drug development began in the early 2000s, initially focusing on bioinformatics and machine learning applications designed to analyze protein structures and identify potential drug candidates.
In 2014, Google DeepMind's AlphaFold made a breakthrough in protein folding prediction, demonstrating the potential of AI in drug discovery.
Since then, the application of AI in drug development has accelerated, with global pharmaceutical companies expanding collaborations with AI platforms to optimize their research efforts and development processes.
Traditionally, identifying new drug candidates has taken several years.
However, AI has dramatically reduced this timeline.
For instance, Insilico Medicine, an AI-based drug development company, successfully identified a drug candidate in just 46 days using AI.
AI also contributes to the ethical aspects of drug development.
By utilizing Simulated Drug Testing technology, AI can predict the biological responses of new drugs, reducing the need for animal testing.
This advancement helps to minimize ethical concerns surrounding animal experimentation while improving research accuracy.
The future of AI in drug development extends beyond drug development to personalized medicine.
AI is being developed to analyze vast datasets, assessing a patient’s genetic profile and medical history to recommend the most effective drug and dosage.
In the near future, AI-powered prescription systems are expected to be widely adopted in hospitals, enabling personalized treatments tailored to each patient's genetic information.
Furthermore, AI is anticipated to play a crucial role not only in drug discovery but also in disease prediction and prevention.
Instead of merely treating diseases after they occur, AI can analyze health data to predict potential future illnesses.
As an illustration, if AI predicts a high risk of heart disease in 10 years, preventive measures such as lifestyle modifications or early medication interventions can be implemented to reduce the likelihood of disease onset.
Leading pharmaceutical and biotech companies are actively integrating AI into drug development to strengthen their research and development capabilities.
Pfizer collaborates with CytoReason to develop AI-driven drug candidates, while Novartis partners with Generate:Biomedicines to advance protein-based therapeutics.
Merck is working with AWS and AstraZeneca to enhance AI-powered drug research.
Moreover, Recursion Pharmaceuticals has secured a $150 million investment for its AI drug discovery platform.
Recursion Pharmaceuticals CEO Chris Gibson described how AI is accelerating their drug development process.
He explained that their AI platform analyzes vast amounts of data every 15 minutes, helping to discover new drug candidates quickly.
One example is their work on a treatment for cerebral cavernous malformation, a rare disease that affects six times more patients in the U.S. than cystic fibrosis.
Gibson emphasized that AI allows Recursion to tackle diseases that other companies avoid due to high costs and risks, offering new hope to many families.
“We’re committed to this work for the next 10 to 20 years,” Gibson stated, underscoring the long-term potential of AI in addressing complex diseases.
Despite the transformative impact of AI on drug development, several challenges remain.
AI models rely on high-quality medical data, but concerns about patient privacy and data accessibility persist.
Pharmaceutical companies require vast medical datasets, yet data security and regulatory constraints limit data sharing and utilization.
Furthermore, AI-driven drug discovery models must demonstrate reliability to gain regulatory approval from key agencies such as the U.S. Food and Drug Administration and the European Medicines Agency, which could introduce additional delays.
Also, AI-generated drugs may pose unforeseen risks, necessitating rigorous validation.
Therefore, human expertise remains essential in AI-assisted drug development, requiring ongoing collaboration, regulatory adaptation, and ethical considerations.
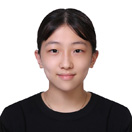
- Esther Kim / Grade 10 Session 9
- Lexington High School