Anthropic takes the very first step in closely investigating the mind of LLMs
[A brain on two sides. Image Credit to Pixabay]
On May 21, Anthropic, a leading AI company and a formidable rival to OpenAI, released groundbreaking research aimed at demystifying the functioning of Large Language Models (LLMs).
Known for its powerful language model, Claude, Anthropic unveiled their findings on their website.
LLMs are essentially large deep learning models, a subset of machine learning, which involves mathematical data analysis processes.
Despite their creators’ understanding of the basic components, the exact workings of LLMs have remained a mystery, akin to understanding the human brain’s structure but not its function - a dilemma often referred to as the “black box problem.”
The black box problem signifies a scenario where input yields a corresponding response without clarity on the underlying reasoning.
Anthropic’s researchers noted that while they can identify which neurons in a model are activated by certain inputs, interpreting the implications of these activations has been challenging.
To resolve this issue, the researchers recalled their previous attempt at applying a technique called “dictionary learning,” derived from classical machine learning, to a very small LLM.
This technique is used to match patterns of activated neurons to human-comprehensible ideas.
In simpler terms, they developed a technique to analyze words and sentences as a whole, rather than examining each individual letter, allowing them to discern hidden meanings or intentions in the text.
They named these matching patterns identified through dictionary learning as “features.”
These features were found to correspond to real-world concepts like DNA sequences or mathematical nouns in smaller models.
Encouraged by these results, Anthropic scaled the technique to larger models, including Claude 3 Sonnet, a state-of-the-art LLM.
The research revealed that features extracted from Claude 3 Sonnet exhibited greater depth and abstraction compared to those from smaller models.
These features corresponded to an immense scale of entities, such as cities, people, atomic elements, scientific fields, and programming syntax.
The features were also multimodal and multilingual, meaning they respond to both images and descriptions in multiple languages.
For example, one identified feature was “the Golden Gate Bridge feature,” which activated in response to descriptions and images of the iconic bridge, across various languages.
The researchers also measured the distance between different features based on specific neuron activation patterns.
This led them to discover an interesting characteristic of the model: features related to the Golden Gate Bridge, such as Alcatraz Island, Ghirardelli Square, and even Alfred Hitchcock’s film Vertigo, were included.
As the researchers expanded such analysis to more features, they found that the model showed a similar organization of concepts to that of humans.
They also learned the ability to adjust model features, amplifying or suppressing them.
When the Golden Gate Bridge feature was amplified, the model responded as if it were the bridge itself, even in unrelated contexts.
This ability to adjust features provided insights into the model’s inner workings and highlighted the potential to enhance model safety by understanding and manipulating these features during the training stage.
However, the researchers emphasized that their work is still preliminary, and additional research is needed to fully understand LLM mechanisms and their safety implications.
Like the efforts of the Anthropic research team, trials aimed at achieving a deeper understanding of large language models have just begun, potentially implying the advent of innovative measures that can also improve the overall safety of these models.
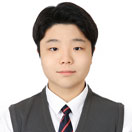
- Huitak Lee / Grade 11
- Korea Digital Media High School